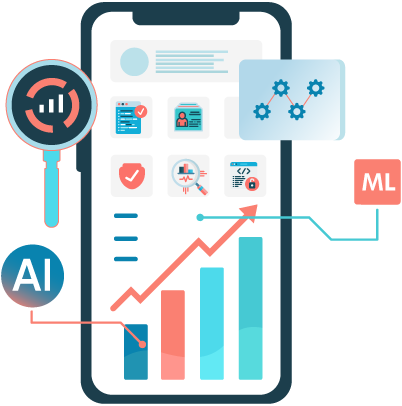
Nowadays, applications are the backbone of businesses across industries, ensuring optimal performance. Application Performance Monitoring (APM) plays a crucial role here, offering invaluable insights into application health, efficiency, and user experience. However, traditional APM often falls short of providing comprehensive visibility and actionable intelligence. Its limitations, such as restricted information, fragmented data interpretation, and reliance on manual application logs, can hinder effective monitoring and analysis.
These challenges require a transformative approach to Artificial Intelligence (AI) and Machine Learning (ML) to monitor application performance. Organizations can extend the boundaries of traditional APM methodologies, unlocking a wealth of possibilities for proactive, predictive, and prescriptive insights.
AI/ML's Impact on Application Performance Monitoring
The application performance monitoring tool's reliance on static thresholds and manual analysis must be improved to accommodate rapidly evolving architectures and user expectations. Traditionally, the tool does not provide end-to-end visibility for fragmented transaction paths, and tracing unstructured data points is time-consuming.
Implementing AI and ML in the APM brings a seismic shift to monitor application performance by integrating advanced analytics engines capable of detecting anomalies, correlating vast datasets in real time, and automating root cause analysis.
Some of the most commonly used application performance monitoring tools, such as New Relic, AppDynamics, and Site 24*7, have integrated AI algorithms and ML models capable of analyzing vast amount of data and extending monitoring capabilities. AI-based tools empower businesses in a way that:
- Transform from reactive to predictive troubleshooting
- It allows businesses to not only react to performance issues but also anticipate and prevent them before they arise
- Deliver unparalleled user experiences, letting you control your application's performance and user satisfaction
AI-based APM Tool Workflow
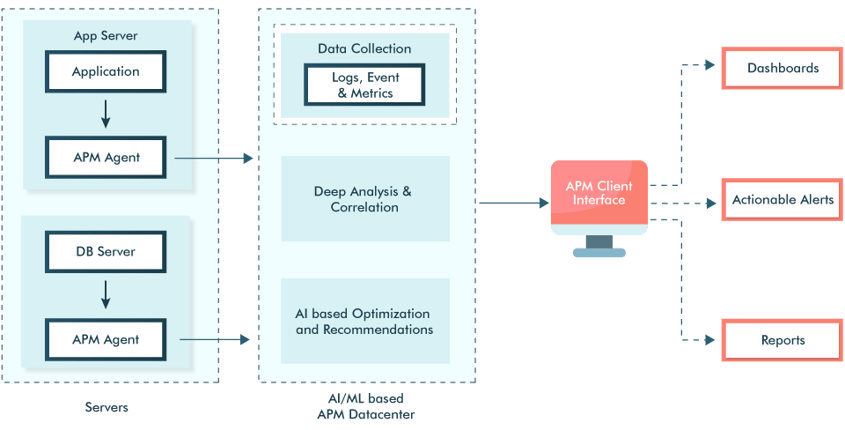
Key Features of AI-based APM Systems
- Correlating metrics with each other to provide actionable insight
- Comprehensive visibility and map interdependencies for deep root cause analysis
- Adjust the warning thresholds dynamically, avoiding alert storms
- Gaining visibility across infrastructure, networks, and user transactions at a granular level
- Autonomously learning each metric behavior
- Pattern analysis for specific components for microservices and containerized environments
Use Cases for AI-based APM Systems in Performance Testing
Below are some use cases of AI/ML application performance monitoring best practices, along with details on the challenges they address and how AI/ML resolves them:
-
Anomaly Detection
Without AI: Identifying abnormal behavior was time-consuming.
With AI: AI/ML helps by automatically flagging deviations as anomalies, enabling proactive issue identification.
-
Root Cause Analysis
Without AI: For complex architecture, identifying the root cause of performance issues requires extensive manual effort.
With AI: AI/ML algorithms analyze correlations between various metrics and events in real-time.
-
Predictive Performance Analysis
Without AI: Performance test engineers manually analyze historical performance data to predict future trends and potential issues.
With AI: AI-enabled APM tools utilize machine learning to analyze historical data, predict performance issues, and prevent downtime, ensuring optimal performance.
-
Dynamic Baseline Generation
Without AI: Establish the performance thresholds manually based on historical data and predefined rules or benchmarks.
With AI: Dynamically learns and adjusts performance baselines using historical data, ensuring alerts are only triggered for genuine anomalies, thereby reducing noise and alert fatigue.
-
User Experience Monitoring
Without AI: Traditional APM tools focus majorly on performance metrics and end-user experience is overlooked.
With AI: AI-based APM incorporates user behavior and sentiment analysis that helps in providing personalized user experiences based on user data from different geolocations and across different platforms like the Web and mobile.
Conclusion
Using AI-based application performance monitoring best practices, one can add value by automating performance monitoring and analysis, predictive maintenance, root cause analysis, and personalized user experiences. While data accuracy, quality, and privacy concerns persist, we anticipate significant advancements in AI-based APM solutions. With ongoing enhancements in machine learning algorithms, monitoring and analyzing performance data will become increasingly accurate and efficient.
The QASource performance testing expert team knows how best to utilize APM tools with the performance testing framework and extend the benefits of AI-enabled APM to help meet projected business outcomes.
Have Suggestions?
We would love to hear your feedback, questions, comments, and suggestions. This will help us to make us better and more useful next time.
Share your thoughts and ideas at knowledgecenter@qasource.com