Integrating cutting-edge technologies has become more crucial as organizations strive for operational efficiency, seamless workflows, and superior product quality. Imagine a scenario where automation isn't just a tool but an intelligent, self-learning entity that continuously adapts, evolves, and perfects itself.
Hyperautomation 2.0 is the vision – a dynamic, modern-day approach that stands tall, surpassing the limitations of traditional QA approaches. Hyperautomation brings together the power of AI, automation, and analytics to revolutionize software testing processes.
The global Hyperautomation market size is estimated at USD 40.96 billion in 2022, and it is expected to hit around USD 197.58 billion by 2032, expanding at a CAGR of 17.04%.
Hyperautomation Market Size 2022 To 2032 (USD Billion)
What Does Hyperautomation 2.0 Bring for ‘Quality Assurance’?
Hyperautomation-driven QA enables organizations to deliver high-quality software quickly and efficiently by automating repetitive tasks, providing intelligent insights, and improving overall test coverage. The following are the major verticals to target using hyperautomation for QA:
- Finance
- Healthcare
- Retail
- Collaboration
- Productivity
However, unlike the conventional reliance on test case scenarios and results analysis, ‘Hyperautomation-led-QA’ aims to automate the end-to-end validation process using core AI technologies. Choosing an Intelligent QA process becomes imperative when striving to meet the following essential requirements:
- Daily or faster release cycles
- Decrement in maintenance efforts
- Improvement in cross-browser testing platforms set
- Levelling up the reporting capabilities
- Getting rid of test flakiness challenges
- Betterment in traceability
Comparison Analysis Between Traditional and Hyperautomation Led QA
Following is the timeframe comparison between traditional and modern QA based on the parameters.
Parameter | Traditional QA | Hyperautomation led QA | Remarks |
---|---|---|---|
Requirements Gathering and Selection
|
Low
|
High
|
Implementation of Intelligent QA requires in-depth knowledge of testing frameworks + AI based technologies. Therefore, selection of the best-suited tools requires appropriate analysis.
|
Script Generation Mode
|
High
|
Low
|
AI driven test automation tools offer higher test coverage and cross-platform scalability, which results in faster release cycles. A few examples are TestRigor, TestSigma, Mabl, and LambdaTest.
|
Time consumption in QA Maintenance tasks
|
High
|
Low
|
Intelligent QA facilitates optimized functions and short algorithms with smart waits, intelligent error handling, and self-healing techniques.
|
Forums and Technical Support
|
High
|
Low
|
‘Hyperautomation in QA’ is still an emerging field, so web-wide support is comparably less than earlier QA techniques.
|
In a digital landscape that demands agility, efficiency, and precision, the Intelligent-QA process driven by Hyperautomation emerges as the catalyst for achieving these essential objectives.
Embedding Hyperautomation 2.0 in QA Workflow
Let’s take a look at the strategies for implanting multiple Hyperautomation-led techniques in the QA cycle:
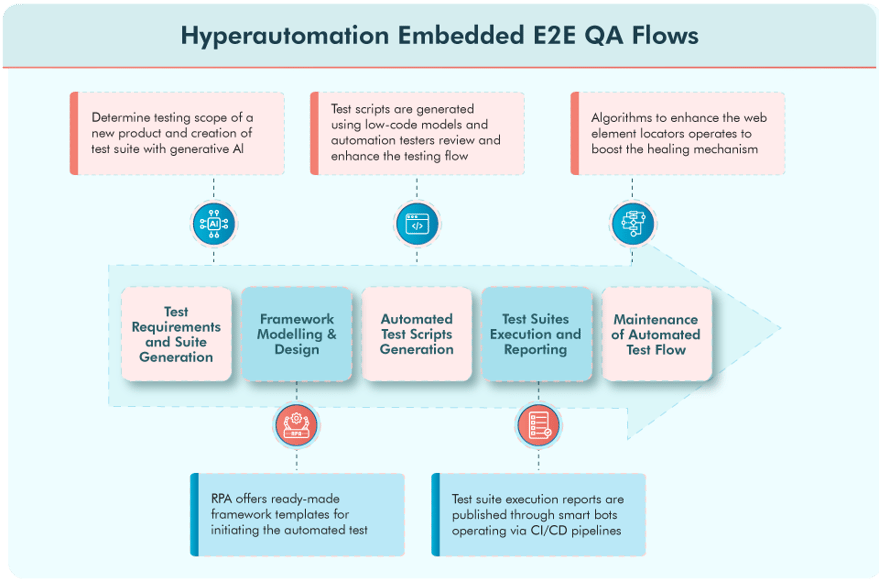
How QASource Embraces QA with Hyperautomation
The following are the few use cases of Hyperautomation in QA:
Self-Adaptive Tests
- Automated QA uses self-healing tests to identify and suggest better alternatives for locator changes.
- Avoid false failure counts in automated tests and simplify test framework maintenance.
- This procedure upscales the reliability and robustness of an automated test framework.
AI-powered Cloud-Based Test Automation
- Testing using cloud-based automated tools eliminates the requirement for hardware maintenance.
- It covers many supported platforms - web, mobile, API, and desktop configurations.
- The tests can be executed in parallel or sequentially by applying grouping.
Digital Twin Testing
- Testing for simulation technology is usually carried out with low-code AI-driven testing methods.
- The QA team also monitors troubleshooting behavior between the real and digital twins.
- The testing environment is intelligently crafted at the client end to validate checkpoints.
Best Practices for Scaling Up with Intelligent QA
Start small and iterate
Define your clear ‘Hyperautomation-led-QA’ objectives. For instance, analyze existing continuous integration flows and define the scope of improvement.
Choosing the right set of tools
Planning and finalizing the desired set of tools per requirement. Key pointers for selecting AI-based tools are ease of use, platform compatibility support, and scalability.
Upscale your team’s skill set
Member’s skill upgrade as per new Intelligent QA demands.
Continuously analysis
Keep documenting details related to progress updates for better analysis.
Evaluate and enhance
Carrying out evaluation checks to make better modifications. Align the automated bots with existing processes.
Wrapping Up
In conclusion, Hyperautomation 2.0 emerges as a powerful force within the QA landscape, revolutionizing the essence of testing methodologies. By intelligently automating scenarios, amplifying test coverage and precision, and collaborating seamlessly, Hyperautomation testing practices are taking new heights. As we witness the convergence of Hyperautomation trends with conventional QA tasks, a comprehensive and end-to-end quality solution comes to fruition. This dynamic blend ensures a smoother transition to the production environment and paves the way for consistently successful software releases.
Have Suggestions?
We would love to hear your feedback, questions, comments, and suggestions. This will help us to make us better and more useful next time.
Share your thoughts and ideas at knowledgecenter@qasource.com