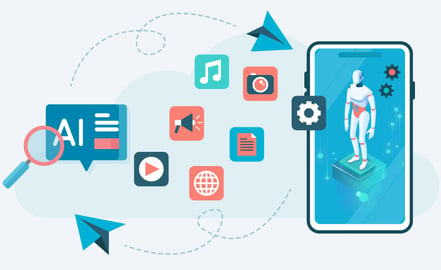
The digital world is continuously evolving, and with this evolution, the concept of superapps has taken center stage. Super apps are multifunctional mobile platforms that integrate a wide range of services, from communication and e-commerce to financial transactions, all within a single application. This blog post delves into what is a superapp, examples, trends, and best practices.
What is a Superapp?
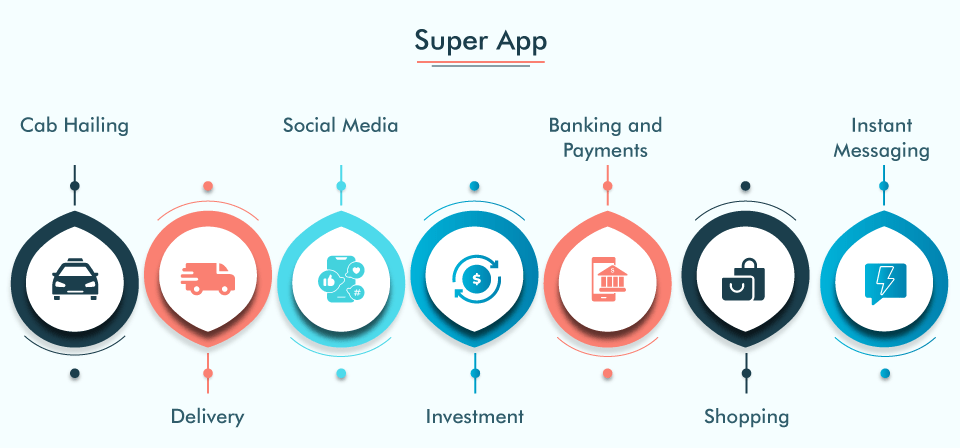
A superapp, also stated as a super app, SuperApp, and super-app, is an all-in-one mobile application consolidating multiple services and functions into a single platform. These services range from messaging and social networking to financial transactions, shopping, and more. Initially popularized in Asia, super-apps offer a streamlined, integrated user experience, reducing the need for multiple standalone apps. Superapps simplify user interaction with technology and are becoming increasingly influential in the global digital landscape by providing a diverse array of services within one app.
SuperApps Architecture
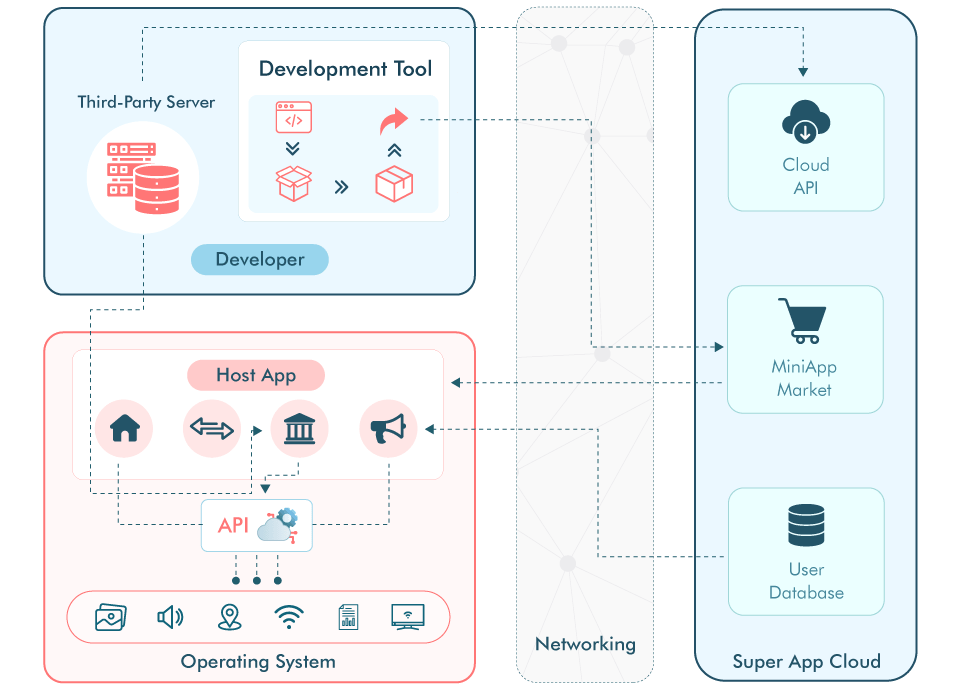
The Evolution of Testing Superapps
The era of super apps demands a testing approach that matches its complexity and dynamism. Traditional manual testing struggles to keep pace with super apps' rapid development and continuous updates. Enter AI, a game-changer that accelerates testing and elevates precision and effectiveness.
Role of AI in Testing Super Apps
As we embrace the power of AI in testing, we witness a paradigm shift where efficiency, accuracy, and innovation converge to propel super apps to new heights.
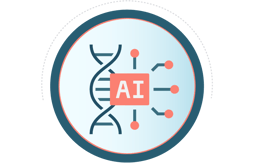
- AI Automation: AI-powered tools are at the forefront of automating the testing process for super apps. It isn't just about saving time and resources but achieving unprecedented accuracy and reliability. With AI handling repetitive and mundane tasks, human testers can focus on more complex and strategic aspects of testing.
- Strategic Test Case Selection: Not all test cases are created equal. AI algorithms introduce a level of strategic thinking into the testing process. By intelligently selecting and prioritizing test cases based on recent changes or updates, AI ensures that testing efforts are concentrated where they matter most. This dynamic approach optimizes resources and guarantees a focused and efficient testing process.
- Self-Healing Tests: AI's contribution goes beyond execution; it extends to resilience. The concept of self-healing tests is a testament to AI's adaptability. These tests dynamically adjust to changes in the application, reducing the need for manual intervention. The result is a testing process that's not only faster but also more robust and resilient to changes.
- Anomaly Detection: Regression issues can be subtle and easily overlooked by traditional testing methods. AI, with its vigilant eye, introduces advanced anomaly detection. This capability is vital to ensuring the super app's reliability and stability.
- Comprehensive Test Data Generation: A comprehensive regression testing strategy requires diverse and dynamic test data. AI steps in to assist with the generation of such data, ensuring that testing covers a wide range of scenarios. It enhances the depth of testing and contributes to the super app's overall robustness, reflecting real-world usage more accurately.
- Performance Regression Testing: AI facilitates performance regression testing by simulating various user loads and conditions. It ensures the super app functions flawlessly and provides an unmatched user experience under different circumstances. AI-driven performance testing is the cornerstone of delivering a seamless and responsive application.
Advantages & Disadvantages
The following are the benefits and challenges associated with superapps, providing a balanced view to understand their impact on software development and testing.
Advantages![]() |
Disadvantages![]() |
---|---|
Convenience | Overwhelming Complexity |
Cost Savings | Dependency Issues |
Enhanced User Engagement | Resource Consumption |
Integrated Ecosystem | Limited Customization |
Efficient Transactions | Market Competition |
Data Synergy | Regulatory Challenges |
Data sharing | Learning Curve |
Future of SuperApps
The global superapps market was worth USD 61.35 billion in 2022 and is predicted to surpass around USD 714.73 billion by 2032, with a CAGR of 27.83% from 2023 to 2032.
SuperApps Market Size, 2022 to 2032 (USD Billion)
Best Practices Followed by QASource in Testing SuperApps
As a software testing organization, there are several best practices and considerations to follow to ensure adequate testing of Super apps with AI. A few are listed below:
Testing Objectives
We clearly define the objectives of AI in testing the superapps, such as determining specific tasks like test automation, anomaly detection, or predictive analysis that can benefit from AI.
Understanding the Complexity of Super-apps
We deeply understand the Superapp's architecture, functionalities, and integration points. Due to this, suitable areas for AI-powered testing can be identified.
Data Quality and Diversity
Subject matter experts and experienced testers concerning the super apps domain are involved in feeding quality data to the AI models for training to cover real-world user cases. This is extremely important to train the models to understand super-apps' behavior better.
Select Appropriate AI Models
Prefer AI models that align with the testing objectives, like machine learning models for predictive analysis, natural language processing for text-based testing, or computer vision for UI testing.
Automation Framework Integration
We are integrating AI into automation frameworks, which aids in achieving end-to-end automation using AI-powered scripts with traditional automation scripts.
Continuous Learning and Adaptation
Prefer the AI models that can learn and adapt over time to help handle the changes in Superapp's features and functionalities. It ensures that AI remains effective in the long term.
AI-Driven Test Data Generation
Use AI to generate diverse and realistic test data that covers main and edge scenarios, which in turn enhances the testing coverage of Super-apps.
Anomaly Detection
Implement AI models to detect anomalies during testing, including the identification of unexpected behaviors, performance bottlenecks, and security vulnerabilities that may not be apparent through traditional testing methods.
Security Considerations
Pay special attention to security testing when using AI, ensure that AI models and training data are secured, and conduct thorough security testing to identify and mitigate any vulnerabilities.
Conclusion
The integration of Artificial Intelligence (AI) into software testing and quality assurance processes marks a significant shift in ensuring application performance and reliability. AI enhances efficiency and accuracy by automating tasks such as test case generation, facilitating better issue detection and prediction. As super apps consolidate various services, they generate a substantial amount of user data. AI can leverage this data to evolve super-apps into personalized digital assistants. Implementing generative AI within superapps, especially for commerce, can elevate the user experience and contribute to business success.
For software testing teams, embracing AI's evolution is crucial. QASource is strategically exploring how AI can revolutionize our approach to testing and quality assurance in the digital age.